Avishek Anand
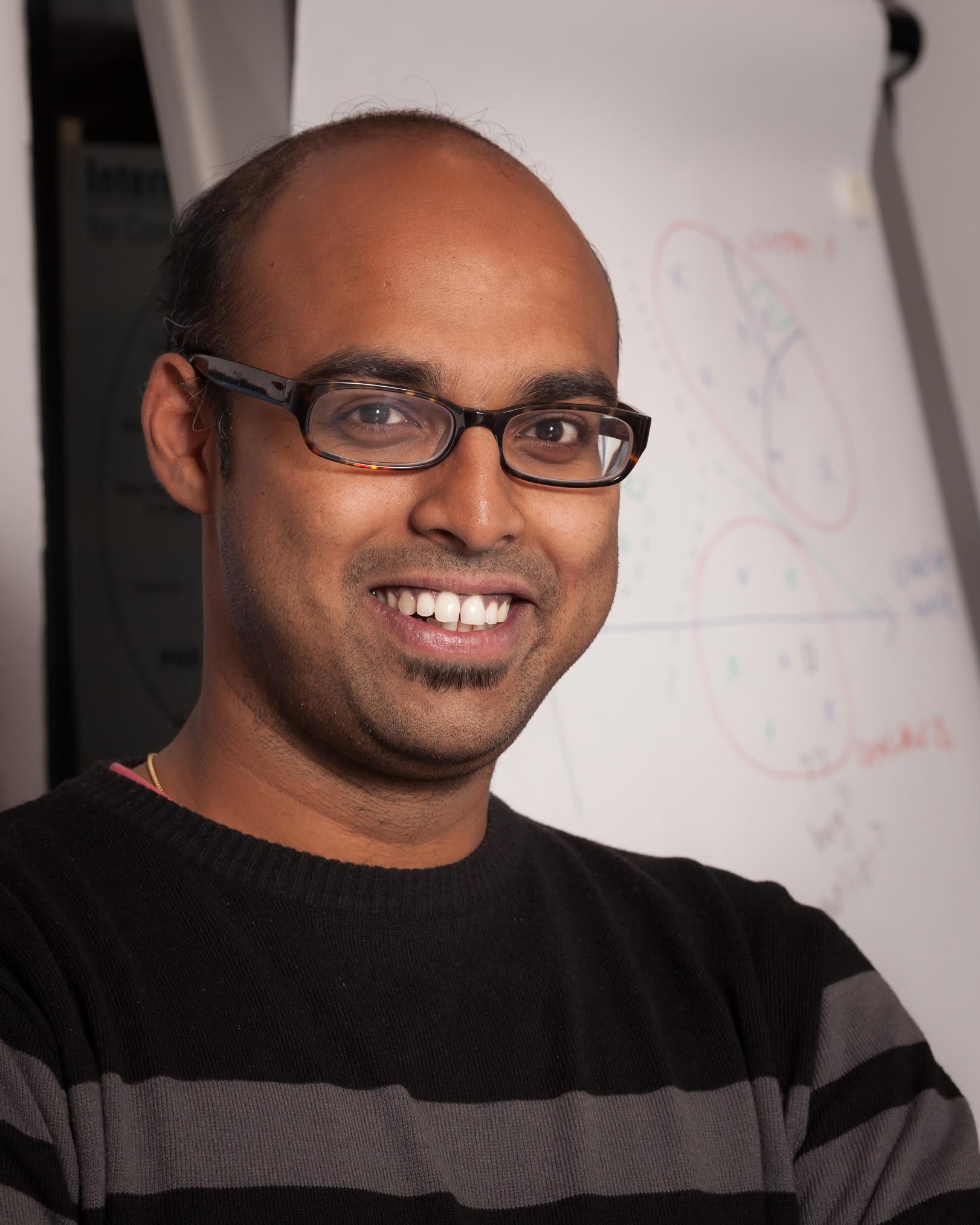
3.West.660, Building 28
TU Delft,
Delft, The Netherlands
Avishek Anand is an Associate Professor in the Web Information Systems (WIS) at the Software Technology department at Delft University of Technology (TU Delft). His research aims to develop intelligent and transparent machine learning approaches to help humans find relevant information. Specifically, he is interested in Explainable Information Retrieval. He holds a PhD in computer science from the Max Planck Insitute for Informatics, Saarbrücken. Previously, he was an Assistant professor in Information Retrieval in Lebniz University Hannover. His research is supported by Amazon research awards, Schufa Gmbh, BMBF, and EU Horizon 2020. He is also a member of the L3S Research Center and was a visiting scholar in Amazon Search. He also leads the Research, Engineering, and Infrastructure Team (REIT) at the Department of Software Technology.
news
Jul 20, 2024 | Paper accepted at ICML 2024, titled “Local Feature Selection without Label or Feature Leakage for Interpretable Machine Learning Predictions”. 🎉 |
---|---|
May 06, 2024 | Paper accepted at the TKDE Journal, titled “DINE: Dimensional Interpretability of Node Embeddings”. Arxiv 🎉 |
Mar 24, 2024 | 🎤 Invited talk on “Explainable Information Retrieval” as part of Data Science Talk Series, Bloomberg, London. |
Dec 24, 2023 | 🏆 Best Paper Award at IEEE International Conference on Data Mining (ICDM) for our paper titled “A Deep Reinforcement Learning Approach to Configuration Sampling Problem”. |
Nov 08, 2023 | 📢 Organized the Dutch-Belgian IR Conference or DIR 2023! Fun talks with some great minds in IR 🌐 🔍 |
selected publications
-
Zorro: Valid, Sparse, and Stable Explanations in Graph Neural NetworksIEEE Trans. Knowl. Data Eng., 2023
-
A Deep Reinforcement Learning Approach to Configuration Sampling ProblemIn IEEE International Conference on Data Mining, ICDM 2023, Shanghai, China, December 1-4, 2023 , 2023
-
Understanding, Categorizing and Predicting Semantic Image-Text RelationsIn Proceedings of the 2019 on International Conference on Multimedia Retrieval, ICMR 2019, Ottawa, ON, Canada, June 10-13, 2019 , 2019